Building a Real-Time AI Voice Agent
Overview
Last year, I embarked on one of the most challenging yet rewarding projects of my career: creating a real-time AI voice agent that could be repurposed across multiple industries like insurance and healthcare. The idea was ambitious—develop an AI that could handle natural conversations, interface seamlessly with custom systems, and scale to manage high call volumes.
I want to share not just the technical aspects but also the personal struggles and victories that came with this journey. If you're interested in AI, voice technology, or just love a good behind-the-scenes tech story, this one's for you.
The Vision and the Challenge
The goal was clear but daunting: build an AI voice agent capable of real-time interaction with customers, adaptable to various industries, and scalable for high call volumes. At the time, companies like ElevenLabs were just entering the scene, offering cutting-edge AI voice solutions. However, latency was the looming challenge. To make conversations feel natural and smooth, the AI needed to respond almost instantaneously—a feat easier said than done.
In human conversations, even a slight delay can make interactions feel awkward or frustrating. I knew that to create a successful AI agent, minimizing latency was non-negotiable. This became the central focus of the project and, admittedly, one of my biggest technical headaches.
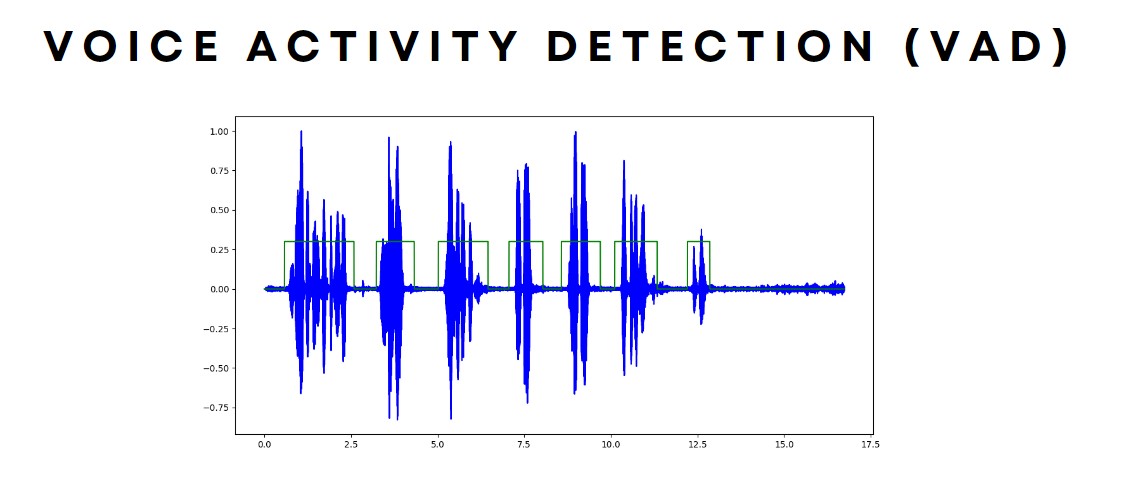
Stage 1: Cracking Voice Detection
WThe first hurdle was determining precisely when a customer had finished speaking so the AI could chime in without interrupting or lagging. Initially, I underestimated how tricky this would be. There were moments when the AI either cut off the customer or left them hanging in awkward silence. After some trial and error, I discovered Silero Voice Activity Detector—a lightweight neural network that could detect speech in 30ms chunks. By monitoring these chunks and setting a threshold (like six consecutive segments of no speech equaling 180ms), the AI could reasonably infer when to respond. If the customer started speaking again, the counter would reset. Technical Tidbit: Adjusting the threshold was a balancing act. Too short, and the AI interrupts; too long, and the conversation feels sluggish. The best solution was to adjust it throughout the call. At the start it was set to just 30ms, and increased further into the call.
Stage 2: Wrestling with Transcription Latency
Transcribing speech in real-time was another significant challenge. Initial tests showed unacceptable delays, making conversations feel robotic. I felt a bit overwhelmed, realizing that our transcription speed could make or break the project. To tackle this, I set up GPU-accelerated servers on AWS, strategically located near our ISP's servers to reduce network latency to under 2ms. We started with OpenAI's Whisper but switched to Faster Whisper to shave off precious milliseconds. We kept the model loaded in memory and adjusted the beam width dynamically. The beam width is equivalent to search space. For common phrases, a smaller beam width sped up processing and gave better results (a win win). For complex or variable inputs like addresses, we increased the beam width to improve accuracy. By this point of the call a little more latency was acceptable.
Stage 3: Crafting the AI's Voice with Language Models
Creating natural and contextually appropriate responses was crucial. We fine-tuned an OpenAI language model and used detailed system prompts to guide its behavior. Surprisingly, this worked exceptionally well. The AI started to respond in ways that felt genuinely human. It was one of those "Eureka!" moments where all the late nights felt worth it. Our next goal was to host an open-source model on our own servers to improve speed and reduce costs. Unfortunately, project funding dried up before we could make this a reality. That was a tough pill to swallow.
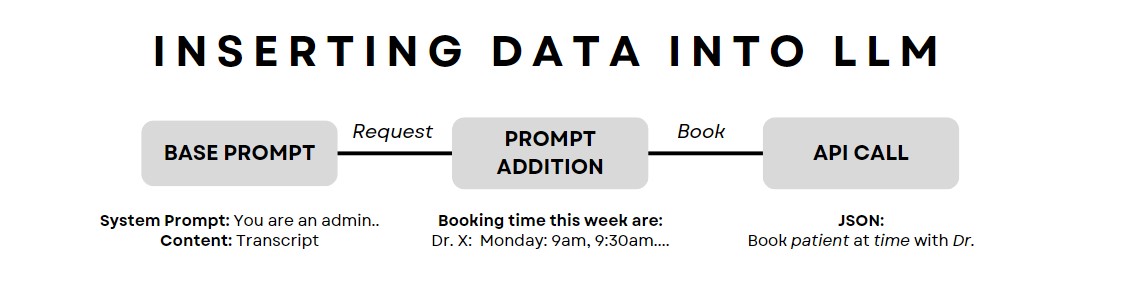
Stage 4: Enabling Actions Through Conversation
We wanted the AI not just to chat but to perform actions like updating records or scheduling appointments. This added a layer of complexity that I found both exciting and intimidating. By training the LLM to include special prompts in its responses (like [update]), we could trigger specific actions behind the scenes. Parsing these prompts allowed us to execute API calls without the customer or LLM knowing.
Technical Insight: This method was surprisingly effective and felt like a neat trick to bridge conversational AI with functional tasks.
Stage 5: Synthesizing Speech Without the Wait
Generating the AI's spoken responses quickly was another hurdle. Any delay here would ruin the conversational flow we had worked so hard to achieve. We decided to cache all generated audio, breaking down responses into sentences to maximize reuse. This approach significantly reduced latency. To do this, we fine-tuned an ElevenLabs model that could output in μ-law format, compatible with our telephony system.
Personal Anecdote: I remember spending an entire day testing different background noises—from office chatter to soft hums. It was a weird but fun detour that paid off.
Stage 6: The Unexpected Nightmare of Telephony Integration
I thought integrating with the telephony system would be straightforward. Boy, was I wrong. The system was outdated, poorly documented, and used a low-bitrate format called μ-law that required precise formatting. We looked at 10 different providers before settling on three for There were days when I felt like I was banging my head against a wall. Minimal logging meant that errors were cryptic, and support was virtually non-existent. Through relentless testing and a bit of reverse engineering, I finally managed to get our audio to interface correctly with the telephony system. It was a massive relief.
Personal Reflection: This stage taught me patience and the importance of not underestimating any part of a project. It's often the "simple" things that trip you up. Quickly testing each part of the system to create a quick working system will help illuminate where the unexpected challenges might come from.
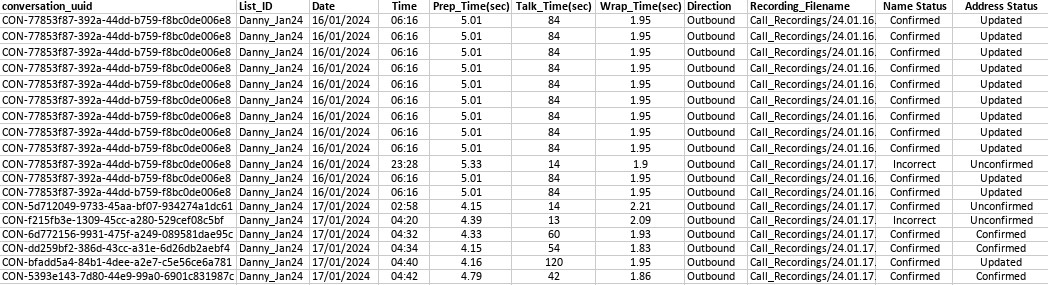
Stage 7: Post-Call Analytics
After each call, we didn't just log the usual metrics. We used a larger, more powerful LLM (GTP 4 at the time) to generate detailed summaries, extracting customer details, actions taken, and even assessing the quality of the interaction. This way we could easily track performance of our system as it scaled and catch errors as they arose. This added layer provided valuable insights and was a fantastic application of LLMs. It felt like we were not just building a tool but creating a system that could learn and improve over time.
Wrapping Up: Lessons Learned and Looking Ahead
This project was a whirlwind of challenges, learning, and personal growth. I delved deep into areas I hadn't touched before, from neural networks for voice detection to the nitty-gritty of telephony systems. What's even more astonishing is how much AI technology has advanced in just the past 6-12 months. If I were to start this project today, I'd approach many aspects differently, leveraging newer technologies that would make it faster and more efficient. This experience reinforced my passion for AI. The rapid advancements mean there's always something new to learn, and the possibilities are virtually endless. I can't wait to dive into the next project and see where this ever-evolving field takes me.